Today, “best practice” models pose more risk than reward. Single Blueprint Technology (SBT) relies on one-size-fits-all methods, overlooking the unique challenges within datasets—resulting in costly errors and misinterpretations.
Data Alchemy (DA) takes a different approach. By adapting to the nuances of each case, DA uncovers hidden risks and builds a stronger foundation for strategic decisions. The following case studies show how DA transformed complex challenges into managerially relevant strategies, while traditional SBT methods fell short.
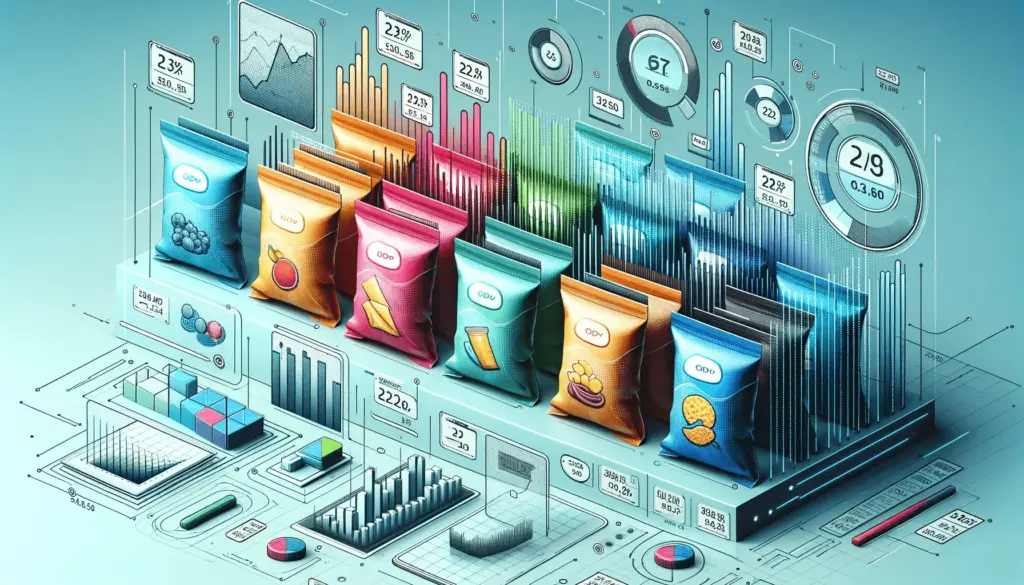
Situation: A snack company needed to adjust prices across a range of products varying by flavor and pack size to maintain profitability amid rising costs.
Complication: Using standard conjoint analysis, they found that pack size and price were confounded, leading to impractical pricing recommendations. The model suggested pricing bulk packs lower than individual packs, creating a credibility risk for decision-makers who couldn’t justify the findings.
Outcome: The DA approach led to adopting Price Pack Architecture-conjoint (PPA-conjoint), which accounted for diminishing utility in larger pack sizes due to factors like storage and portion control preferences. This DA-driven approach eliminated confounding, leading to a sensible pricing strategy that aligned with consumer behavior and safeguarded the research team’s credibility with reliable, business-relevant recommendations.
Situation: The video game industry wants to optimize revenue by understanding player preferences for game modes, such as “Capture the Flag,” offered as part of the base game, downloadable content, or a subscription.
Complication: Using standard conjoint analysis, they encountered multicollinearity issues as game modes appeared in multiple formats. Multiple parameters for each game mode created a statistical confusion, leading to inconsistent outputs that misinterpreted player preferences—posing a risk of unreliable recommendations that could mislead pricing strategy.
Outcome: DA inspired a custom model that identified each game mode across formats, introducing a parameter to measure preferences based on delivery method. This approach resolved multicollinearity, delivering coherent insights and empowering decision-makers with reliable, profit-optimized strategies.
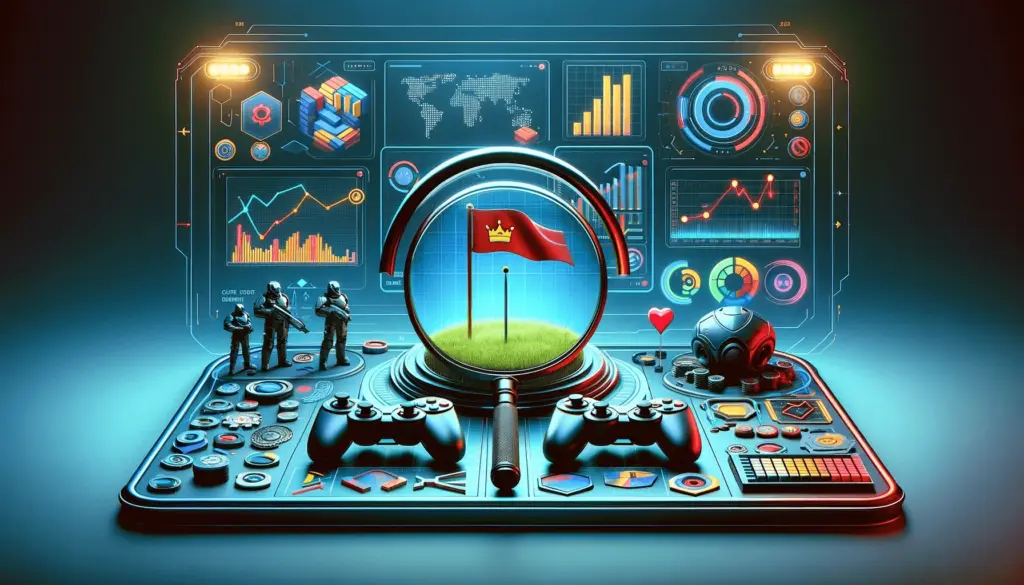
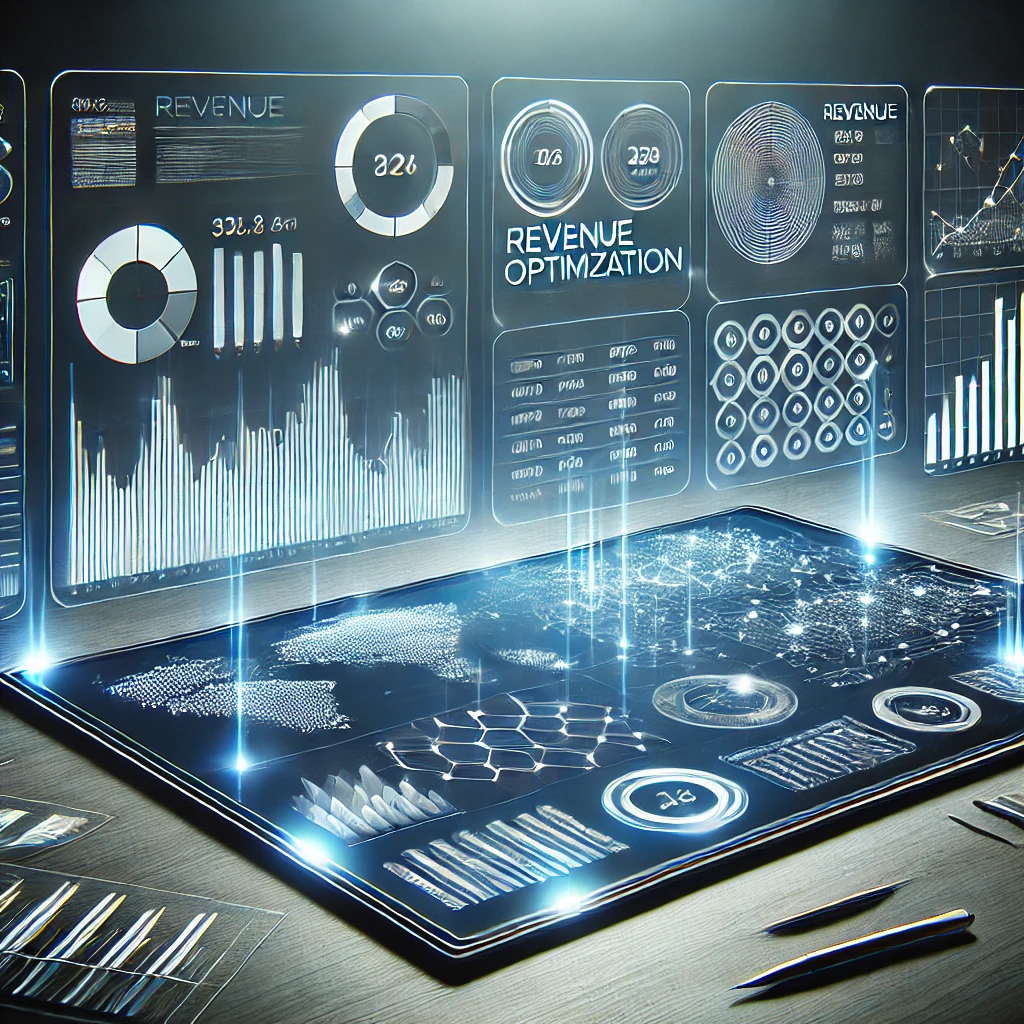
Situation: A software company aimed to use conjoint analysis to guide its portfolio pricing strategy, seeking detailed insights to inform product decisions.
Complication: Mid-project, they found that the standard conjoint software couldn’t produce the needed outputs due to an incompatibility with the study design. When they contacted the software provider, a code rewrite was off the table. This left the company at risk of basing decisions on generic “importance” metrics, lacking the depth for true portfolio-level insights.
Outcome: DA provided a customized model and simulator that resolved the software incompatibility, enabling the shift from basic importance scores to portfolio-level revenue optimization. This solution equipped the company to make strategic, data-driven pricing decisions with confidence.
Talk About Your Upcoming Project?
Interested in seeing how Data Alchemy can drive precise, strategic insights for your clients? Schedule a strategy meeting to explore custom analytics solutions tailored to your unique challenges.